
Image credit: Nils B. Tack
Status: Active August 2024 – present
​
PI: Prof. Monica Wilhelmus (Brown University)
Metachronal robotics
My current postdoctoral work with Prof. Monica Wilhelmus involves developing and controlling swarms of autonomous shrimp-inspired robots for ocean exploration. I utilize tools of fluid mechanics to uncover the physics of multi-legged propulsion, soft robotics for designing a scalable and practical ten-legged mechanism, and machine learning to optimize appendage kinematics. Ultimately, this work lays the groundwork for the deployment of swarms of highly agile and maneuverable ocean-exploring robots, while also improving our understanding of shrimp-like propulsion as it appears across a vast number of species in the ocean.

Status: Active April 2024 – present
PI: Prof. John Dabiri (Caltech)
Surfing vortex rings for energy efficient propulsion
Leveraging background fluid flows for propulsion has the potential to enhance the range and speed of autonomous aerial and underwater vehicles. In this work, we demonstrated experimentally a fully autonomous strategy for exploiting vortex rings for energy-efficient propulsion. First, an underwater robot used an onboard inertial measurement unit (IMU) to sense the motion induced by the passage of a vortex ring generated by a thruster in a 13,000-liter water tank. In response to the sensed acceleration, an impulsive maneuver entrained the robot into the material boundary of the vortex ring. After entrainment, the robot was propelled across the tank without expending additional energy or control effort. By advecting with the vortex ring, the robot achieved a near five-fold reduction in the energy required to traverse the tank. This study serves as a proof-of-concept demonstration of the potential for onboard inertial measurements to enable efficient interaction with background fluid flows (Gunnarson & Dabiri, in review).
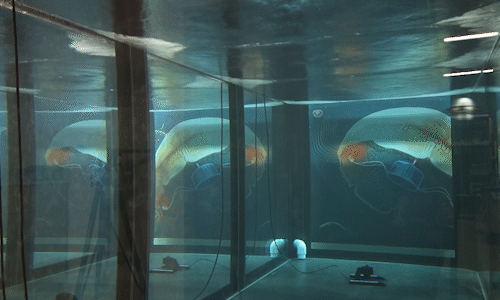
Status: Active December 2021 – March 2024
PI: Prof. John Dabiri (Caltech)
Tracking underwater turbulent plumes with RL and fish-inspired pressure sensors
Inspired by the ability of aquatic animals to navigate via flow sensing, this work investigates hydrodynamic cues for accomplishing targeted sampling using Reinforcement Learning (RL). We equipped a palm-sized robotic swimmer with distributed pressure sensors to mimic the function of canal neuromasts in the lateral line of fishes. All aspects of the robot are scratch built, including custom RL and neural network libraries that enable real-time training of a deep neural network using only a megabyte of onboard memory and half a watt of power consumption. As a model navigation problem, we tasked the robot with navigating towards a turbulent jet plume in a six by six by sixteen-foot water tank. Using data collected from an hour of swimming around in the tank, the RL algorithm discovered a simple navigation policy for finding the jets using a velocity gradient measurement (Gunnarson & Dabiri, 2024).
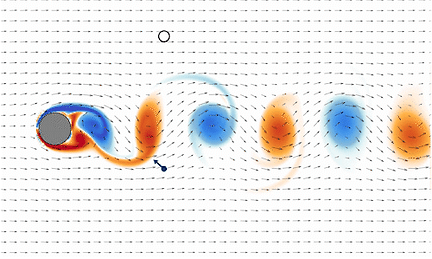
Status: Active May 2020 – December 2021 (simulated studies); ongoing project implementing these results in a robotic platform
PI: Prof. John Dabiri (Caltech)
Collaborators: Prof. Petros Koumoutsakos, Dr. Guido Novati, and Ioannis Mandralis (ETH Zurich)
Autonomous Navigation in Simulated Flow with Reinforcement Learning
Efficient navigation in the presence of background ocean currents or wind gusts is a challenge in many robotic applications, including ocean exploration, monitoring deep-sea animal communities, and drone-based inspection. In such applications, the background flow is typically unknown, difficult to measure, and time varying. As a result, oceanic and aerial robots may only have knowledge of their immediate surroundings from onboard sensors, which limits the use of optimal control theory for planning efficient trajectories through the global flow field. A bio-inspired approach is to navigate using local flow information. For example, sea lions sense hydrodynamic cues of prey to hunt in the dark, and zebrafish sense flow gradients with their lateral line to avoid running in to walls. In this work, we collaborated with Professor Koumoutsakos and his students to use RL to compare flow sensing strategies for navigating in a 2D, unsteady simulated flow field. We found that deep RL can discover time-efficient, robust navigation policies by sensing local flow information, reaching target locations in the flow that are unreachable by simpler strategies such as swimming directly towards the target (Gunnarson et al., 2021).
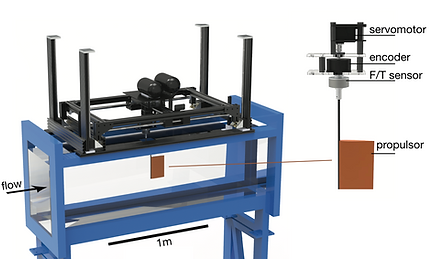
Status: Active June 2018 – June 2019
PI: Prof. Dan Quinn (University of Virginia)
Collaborators: Dr. Qiang Zhong (University of Virginia)
Modeling Bio-Inspired Propulsion for Maneuvering
Fish use asymmetric flapping motions to execute rapid turning maneuvers for predator escape, avoiding obstacles, and for station keeping. The hydrodynamics behind these maneuvers are an important driver for fish morphology but are poorly understood because classic analytical models for oscillating hydrofoils are derived under the assumption that the flapping motion is symmetric. To investigate maneuvering experimentally, we measured the forces and trajectories of a flapping hydrofoil in a water channel. The foil was mounted using air bearings for near-frictionless movement, and actuated with a servo motor that could be controlled to execute maneuvers. We found that classic theories of flapping hydrofoils greatly under predict the turning force of the asymmetrically flapping hydrofoil. However, by combining the unsteady contribution of the classical theories with empirical force measurements in static thrust tests, we were able to accurately model and predict the trajectory of the flapping airfoil and steer the foil to waypoints around the water channel (Gunnarson et al., 2019).